Wenting LiPostdoc T-5/CNLS Physics-informed learning for Power Grids 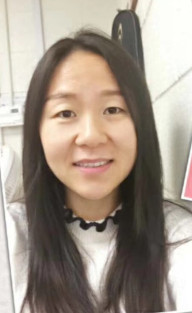
Office: TA-03, Building 0410, Room 163 Mail Stop: B258 Phone: (518) 268-0401 Fax: (505) 665-7652 wenting@lanl.gov home page Research highlight- My general research area is the fusion of machine/deep learning with dynamic physical networks. Particularly, I am interested in distilling information from high-dimensional structured data, sparse networks and fomulating real-time efficient algorithms. Specific applications include event identification, faults location, detection, and non-intrusive load disaggregation in power grids.
|  | Educational Background/Employment:- Ph.D., Electrical Engineering, Rensselaer Polytechnic Institute (RPI), Troy, NY, 2019
- M.S., Applied Mathematics, Rensselaer Polytechnic Institute (RPI), Troy, NY, 2019
- B.S., Electrical Engineering, Harbin Institute of Technology (HIT), Harbin, China, 2013
- Employment:
- 2019-Present: Postdoc Research Associate, Los Alamos National Laboratory, NM
- 2015-2019: Research Assistant, Rensselaer Polytechnic Institute (RPI), Troy, NY
Research Interests: - Physics-informed machine/deep learning for the large-scale dynamic networks
- Real-time algorithm with low-rank sparse data
- Graph-based learning and neural networks
Selected Recent Publications: - Li, W., Yi, M., Wang, M., et al, Real-time Energy Disaggregation at Substations with Behind-the-Meter Solar Generation. accepted by IEEE Transactions on Power Systems, (2020).
- Li, W., Deka, D., Chertkov, M. and Wang, M., Real-time faulted line localization and pmu placement in power systems through convolutional neural networks. IEEE Transactions on Power Systems, 34.6 4640-4651(2019).
- Li, W., Wang, M., Identifying overlapping successive events using a shallow convolutional neural network. IEEE Transactions on Power Systems, 34.6 4762-4772 (2019).
- Li, W., Wang, M. and Chow, J.H., Real-time event identification through low-dimensional subspace characterization of high-dimensional synchrophasor data. IEEE Transactions on Power Systems, 33.5 4937-4947 (2018).
|