Amy LovellPostdoc CNLS/T-2 Nuclear reaction theory 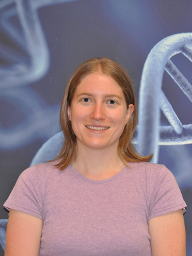
Office: TA-03, Bldg 1690, Room 119 Mail Stop: B258 Phone: (505)606-0966 Fax: (505) 665-2659 lovell@lanl.gov home page Research highlight- Uncertainty quantification for nuclear theory models
- Comparison of uncertainty quantification methods
- Improving the description of optical model potentials
- Machine learning for parametric optimization
- Utilizing correlated fission observables
|  | Educational Background/Employment:- Ph.D. (2018) Nuclear Physics, Michigan State University
- M.S. (2015) Physics, Michigan State University
- B.S. (2013) Physics, Rensselaer Polytechnic Institute
- B.S. (2013) Mathematics, Rensselaer Polytechnic Institute
- Employment:
- May 2018-Present Postoctoral Research Assosiate, T-2/CNLS, Los Alamos National Laboratory
Research Interests: All nuclear theory models contain uncertainties from a variety of sources, including a reduction of the model space, numeric and model approximations, and the use of model parameters that must be constrained by experimental data. These introduce uncertainties in the final calculations that should be rigorously quantified. In particular, Bayesian methods have become popular recently and have been used to quantify uncertainties coming from many of these sources, including those due to the fitting of phenomenological potentials between projectiles and targets to experimental data (the focus of part of my dissertation work). I am further interested in using a variety of optimization techniques to construct global optimizations across the nuclear chart.
Since starting my post-doc at LANL, I have become interested in fission models, in particular understanding and utilizing correlations between observables to extract information indirectly (e.g. extracting neutron multiplicity information from calculations/measurements of gamma-rays and fission fragment energies).
I am also interesting in using machine learning to make predictions for fission models as a way to both increase the speed of calculations and interpolate/extrapolate from sparse experimentally measured data.
Selected Recent Publications: - A.E. Lovell and F.M. Nunes, Constraining transfer cross sections using Bayes' theorem, Phys. Rev. C. 97,064612 (2018).
- A.E. Lovell S. Srinivasan, S. Karra, D. O'Malley, N. Makedonska, H.S. Viswanathan, G. Srinivasan, J.W. Carey, and L.P Frash, Extracting Hydrocarbon From Shale: An Investigation of the Factors That Influence the Decline and the Tail of the Production Curve, Water Resources Research 54,3748 (2018).
- A.E. Lovell, P.-L. Bacq, P. Capel, F.M. Nunes, and L.J. Titus, Energy dependence of nonlocal optical potentials, Phys. Rev. C. 96,051601(R) (2017).
- A.E. Lovell, F.M. Nunes, and I.J. Thompson, Three-body model for the two-neutron emission of 16Be, Phys. Rev. C. 95,034605 (2017).
- A.E. Lovell, F.M. Nunes, J. Sarich, and S.M. Wild, Uncertainty quantification for optical model parameters, Phys. Rev. C. 95,024611 (2017).
- A.E. Lovell and F.M. Nunes, Systematic uncertainties in direct reaction theories, J. Phys. G: Nucl. and Part. Phys. 42,034014 (2015).
|